Introduction:
As technology continues to advance, it is revolutionizing various industries, and one area that has greatly benefitted is the delivery of quality products and services. Artificial intelligence (AI) has emerged as a powerful tool in ensuring the highest standards of quality across different sectors. From manufacturing and healthcare to finance and retail, AI is transforming the way organizations ensure quality control and achieve customer satisfaction. In this blog, we will explore how AI is contributing to the delivery of quality in various industries.

Automated Quality Control:
One of the primary uses of AI in ensuring quality is through automated quality control processes. Traditionally, quality control required manual inspection, which was time-consuming and prone to human errors. However, AI-powered systems can now analyze large volumes of data at high speeds, identifying defects or inconsistencies in real-time. Machine learning algorithms enable AI systems to learn from past data and make precise decisions, reducing errors and boosting efficiency. This not only enhances the overall quality of products but also saves time and resources.
Predictive Maintenance:
Another area where AI is revolutionizing quality delivery is through predictive maintenance. With AI-powered systems, organizations can monitor and analyze data from various sensors and devices to detect potential issues or failures before they occur. This proactive approach allows for timely maintenance, preventing product defects or breakdowns. By ensuring optimal performance and reliability, organizations can deliver high-quality products and minimize customer complaints or returns.
Enhanced Customer Experience:
AI is also contributing to delivering quality through enhanced customer experience. Chatbots and virtual assistants powered by AI algorithms can provide instant support and assistance to customers, ensuring timely responses to their queries. The ability of AI systems to understand and respond to customer needs and preferences contributes to personalized experiences and improves overall customer satisfaction. Furthermore, AI algorithms can analyze customer data to identify patterns, enabling organizations to offer personalized recommendations and anticipate customer requirements accurately.
Productivity and Efficiency Improvements:
By significantly reducing manual work and repetitive tasks, AI improves productivity and efficiency in quality delivery. AI-powered systems can automate various processes, such as data analysis, reporting, and decision-making, allowing quality control teams to focus on more complex and critical tasks. This boosts productivity and eliminates the possibility of human errors, ensuring consistent and high-quality output.
Real-time analytics and Decision-making
AI can be deployed for real-time analytics and decision-making in manufacturing quality through the following methods:
Sensor Data Analysis:
AI algorithms can process data collected from various sensors installed throughout the manufacturing process. These sensors monitor factors like temperature, pressure, humidity, vibration, and other critical parameters. AI can analyze this data in real-time to identify patterns, detect anomalies, and provide insights into the production process's health and quality.
Predictive Analytic:
By employing machine learning models, AI can predict potential quality issues before they occur. These models use historical data and sensor inputs to anticipate deviations or defects, enabling proactive interventions to prevent the production of subpar products.
Automated Quality Control:
AI-powered vision systems can inspect products on the assembly line and compare them to pre-defined quality standards. Any deviations or defects can be identified immediately, triggering an automated rejection or routing the product for further analysis or correction.
Defect Detection and Classification:
AI can recognize and classify defects in real-time, even in complex and high-speed manufacturing environments. This helps in immediate isolation of faulty products and reduces the chances of defective items reaching the end customers.
Predictive Maintenance:
AI can monitor the health of manufacturing equipment and machinery using sensor data. By analyzing the data, AI can predict when maintenance is needed, reducing the risk of equipment failure and avoiding potential defects caused by faulty machines.
Real-time Production Optimization:
AI can continuously analyze data from various stages of production and optimize the process to maintain consistent quality levels. For instance, it can adjust parameters like production speed, raw material usage, or energy consumption to ensure product quality remains within desired specifications.
Dynamic Resource Allocation:
AI can optimize resource allocation in real-time based on current demand, production status, and available inventory. This helps ensure the right resources are allocated to the right tasks, reducing waste and enhancing production quality.
Decision Support Systems:
AI can assist human decision-makers by providing real-time insights and recommendations. Through dashboards and alerts, AI can flag potential quality issues, suggest corrective actions, and help managers make informed decisions on the shop floor.
By integrating AI-driven real-time analytics and decision-making into manufacturing processes, companies can gain a competitive advantage by producing higher-quality products, reducing defects, and increasing overall productivity and efficiency. AI's ability to process vast amounts of data quickly and make data-driven decisions enables manufacturers to respond rapidly to changes and challenges, leading to improved manufacturing quality and customer satisfaction.
Root Cause Analysis
AI can be effectively deployed in manufacturing quality for root cause analysis using the following approaches:
Data Collection and Integration:
The first step is to gather data from various sources within the manufacturing process, such as sensors, production logs, quality control data, and historical records. This data may include information on parameters like temperature, pressure, humidity, machine settings, production time, and more. Integrating and organizing this diverse data is crucial for accurate root cause analysis.
Data Preprocessing:
Before feeding the data into AI algorithms, it needs to undergo preprocessing to clean and prepare it for analysis. This involves handling missing values, normalizing data, and removing outliers that could skew the analysis results.
Machine Learning Models:
AI employs machine learning models to analyze the collected and preprocessed data. Supervised learning algorithms, such as decision trees, random forests, or support vector machines, can be used to classify defect categories or identify fault patterns when labeled data is available.
Unsupervised Learning:
In cases where labeled data is limited, unsupervised learning techniques like clustering can be applied to identify patterns or group similar instances together based on similarities in their attributes. This may help reveal hidden relationships or possible root causes.
Deep Learning:
Deep learning models, such as Convolution Neural Networks (CNNs) and Recurrent Neural Networks (RNNs), can be employed to analyze complex and sequential data. For instance, in the case of video data from manufacturing processes, CNNs can detect patterns related to defects.
Time-Series Analysis:
For processes with time-dependent data, time-series analysis techniques can be used to identify trends, cyclic patterns, and anomalies that may be indicative of root causes.
Model Interpretability :
It's essential to choose AI models that offer interpretability. Interpretable AI models allow engineers and domain experts to understand how the model arrived at its conclusions, making it easier to identify the most influential factors contributing to a quality issue.
Feedback Loop and Continuous Improvement:
The root cause analysis process should be iterative. As new data becomes available, the AI models can be continuously updated to improve accuracy and adapt to changing manufacturing conditions.
Expert Collaboration:
While AI can provide valuable insights, it's essential to involve domain experts and engineers in the process. Their expertise can validate AI-generated conclusions, provide context, and offer additional insights that AI might not capture.
Integrated Decision Support:
Root cause analysis should not be isolated from decision-making. By integrating AI-generated root cause insights into the decision support systems, manufacturers can take prompt corrective actions and prevent further occurrences of quality issues.
The successful deployment of AI for root cause analysis in manufacturing quality requires a combination of data-driven AI techniques, human expertise, and a commitment to continuous improvement. By harnessing the power of AI in this area, manufacturers can improve product quality, reduce defects, and optimize production processes for better overall efficiency.
Continuous Improvement:
AI not only empowers organizations to deliver quality products and services but also enables continuous improvement. By analyzing vast amounts of data, AI algorithms can identify patterns, detect anomalies, and provide insights for process optimization. This feedback loop helps organizations identify areas for improvement, make data-driven decisions, and refine their quality control processes over time.
Conclusion:
Artificial intelligence is transforming the delivery of quality across industries, improving efficiency, productivity, and customer satisfaction. From automated quality control and predictive maintenance to enhanced customer experiences and continuous improvement, AI is revolutionizing how organizations achieve and maintain high-quality standards. As organizations embrace AI technology, they can better adapt to evolving customer needs, stay ahead of the competition, and drive success in the era of digital transformation. With AI's potential for innovation and continuous learning, we can expect further advancements in quality delivery in the coming years, benefiting both businesses and consumers.
Written by:
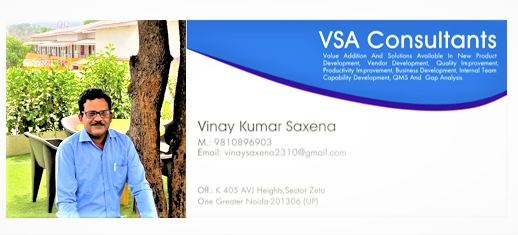
Wonderful 👌👌
AI and Digitisation shall go hand in hand to have gr8 success
Very nice keep it up
Mr. Vinay, I have just gone through this article n got to know the power of AI in modern Quality Excellence field. Indeed AI is a great relief for modern day quality professionals. Please Keep positive such wonderful articles. Kudos 👏
Its wonder full,
uses of AI in RCA would be great
Wonderful !!! Got insights about the near future AI , a very informative blog,got to know a lot about AI and it's application...
Kudos to you Sir 🙌 ....